Summary: Researchers made a significant leap in artificial intelligence by developing an AI capable of learning new tasks from verbal or written instructions and then verbally describing these tasks to another AI, enabling it to perform the same tasks. This development highlights a unique human-like ability in AI for the first time—transforming instructions into actions and communicating these actions linguistically to peers.
The team used an artificial neural model connected to a pre-trained language understanding network, simulating the brain’s language processing areas. This breakthrough not only enhances our understanding of the interaction between language and behavior but also holds great promise for robotics, envisioning a future where machines can communicate and learn from each other in human-like ways.
Key Facts:
- Human-Like Learning and Communication in AI: The University of Geneva team has created an AI model that can perform tasks based on verbal or written instructions and communicate these tasks to another AI.
- Advanced Neural Model Integration: By integrating a pre-trained language model with a simpler network, the researchers simulated human brain areas responsible for language perception, interpretation, and production.
- Promising Applications in Robotics: This innovation opens up new possibilities for robotics, allowing for the development of humanoid robots that understand and communicate with humans and each other.
Source: University of Geneva
Performing a new task based solely on verbal or written instructions, and then describing it to others so that they can reproduce it, is a cornerstone of human communication that still resists artificial intelligence (AI).
A team from the University of Geneva (UNIGE) has succeeded in modelling an artificial neural network capable of this cognitive prowess. After learning and performing a series of basic tasks, this AI was able to provide a linguistic description of them to a ‘‘sister’’ AI, which in turn performed them.
These promising results, especially for robotics, are published in Nature Neuroscience.
Performing a new task without prior training, on the sole basis of verbal or written instructions, is a unique human ability. What’s more, once we have learned the task, we are able to describe it so that another person can reproduce it.
This dual capacity distinguishes us from other species which, to learn a new task, need numerous trials accompanied by positive or negative reinforcement signals, without being able to communicate it to their congeners.
A sub-field of artificial intelligence (AI) – Natural language processing – seeks to recreate this human faculty, with machines that understand and respond to vocal or textual data. This technique is based on artificial neural networks, inspired by our biological neurons and by the way they transmit electrical signals to each other in the brain.
However, the neural calculations that would make it possible to achieve the cognitive feat described above are still poorly understood.
‘‘Currently, conversational agents using AI are capable of integrating linguistic information to produce text or an image. But, as far as we know, they are not yet capable of translating a verbal or written instruction into a sensorimotor action, and even less explaining it to another artificial intelligence so that it can reproduce it,’’ explains Alexandre Pouget, full professor in the Department of Basic Neurosciences at the UNIGE Faculty of Medicine.
A model brain
The researcher and his team have succeeded in developing an artificial neuronal model with this dual capacity, albeit with prior training. ‘
‘We started with an existing model of artificial neurons, S-Bert, which has 300 million neurons and is pre-trained to understand language. We ‘connected’ it to another, simpler network of a few thousand neurons,’’ explains Reidar Riveland, a PhD student in the Department of Basic Neurosciences at the UNIGE Faculty of Medicine, and first author of the study.
In the first stage of the experiment, the neuroscientists trained this network to simulate Wernicke’s area, the part of our brain that enables us to perceive and interpret language. In the second stage, the network was trained to reproduce Broca’s area, which, under the influence of Wernicke’s area, is responsible for producing and articulating words. The entire process was carried out on conventional laptop computers. Written instructions in English were then transmitted to the AI.
For example: pointing to the location – left or right – where a stimulus is perceived; responding in the opposite direction of a stimulus; or, more complex, between two visual stimuli with a slight difference in contrast, showing the brighter one. The scientists then evaluated the results of the model, which simulated the intention of moving, or in this case pointing.
‘‘Once these tasks had been learned, the network was able to describe them to a second network – a copy of the first – so that it could reproduce them. To our knowledge, this is the first time that two AIs have been able to talk to each other in a purely linguistic way,’’ says Alexandre Pouget, who led the research.
For future humanoids
This model opens new horizons for understanding the interaction between language and behaviour. It is particularly promising for the robotics sector, where the development of technologies that enable machines to talk to each other is a key issue.
‘‘The network we have developed is very small. Nothing now stands in the way of developing, on this basis, much more complex networks that would be integrated into humanoid robots capable of understanding us but also of understanding each other,’’ conclude the two researchers.
About this AI research news
Author: Antoine Guenot
Source: University of Geneva
Contact: Antoine Guenot – University of Geneva
Image: The image is credited to Neuroscience News
Original Research: Open access.
“Natural Language Instructions Induce Compositional Generalization in Networks of Neurons” by Alexandre Pouget et al. Nature Neuroscience
Abstract
Natural Language Instructions Induce Compositional Generalization in Networks of Neurons
A fundamental human cognitive feat is to interpret linguistic instructions in order to perform novel tasks without explicit task experience. Yet, the neural computations that might be used to accomplish this remain poorly understood. We use advances in natural language processing to create a neural model of generalization based on linguistic instructions.
Models are trained on a set of common psychophysical tasks, and receive instructions embedded by a pretrained language model. Our best models can perform a previously unseen task with an average performance of 83% correct based solely on linguistic instructions (that is, zero-shot learning).
We found that language scaffolds sensorimotor representations such that activity for interrelated tasks shares a common geometry with the semantic representations of instructions, allowing language to cue the proper composition of practiced skills in unseen settings.
We show how this model generates a linguistic description of a novel task it has identified using only motor feedback, which can subsequently guide a partner model to perform the task.
Our models offer several experimentally testable predictions outlining how linguistic information must be represented to facilitate flexible and general cognition in the human brain.
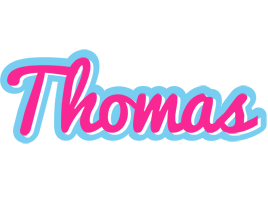
Dr. Thomas Hughes is a UK-based scientist and science communicator who makes complex topics accessible to readers. His articles explore breakthroughs in various scientific disciplines, from space exploration to cutting-edge research.