Overview
We used repeated census data from 23 large forest sites around the globe (Fig. 1) to analyse latitudinal patterns in stabilizing CNDD following a three-step approach. First, we fitted species-site-specific mortality models from repeated observations of individual trees. Second, we used these models to quantify CNDD for each species and site using an estimator designed to maximize robustness, comparability and relevance for fitness and stabilization. Third, we used meta-regressions to consider three distinct latitudinal patterns in CNDD derived from the hypothesis that CNDD is more influential for maintaining local tree species diversity in the tropics. Robustness of the analysis pipeline was validated by model diagnostics and randomization.
This approach is based on recently developed best-practice statistical methods for estimating CNDD. Crucially, the use of dynamic mortality data allowed us to avoid the statistical pitfalls of previous CNDD studies, in particular with regard to analyses of the static relationship of number of saplings to number of adults, in which the null hypothesis is a positive linear relationship but regression dilution flattens this relationship and thus biases analyses towards finding CNDD, especially for rare species10,11,12,28,29. By fitting mortality models in which the null hypothesis is no relationship between survival and number of conspecific neighbours, we ensure that any regression dilution has a conservative effect by reducing CNDD estimates. We also addressed other previously identified limitations of CNDD analyses; namely, nonlinear and saturating CNDD (see ‘Species-site-specific mortality models’), the comparability of CNDD among species and sites (see ‘Quantification of conspecific density dependence’) and the extent to which CNDD estimates are meaningful for stabilization and species coexistence10,25,31.
All analyses were conducted in R v.4.2.1 (ref. 51).
Forest data
The data used in this study were collected at 23 sites with permanent forest dynamics plots that are part of the Forest Global Earth Observatory network (ForestGEO)30 (Fig. 1 and Supplementary Notes), in which all free-standing woody stems with a diameter of at least 1 cm at 1.3 m from the ground (DBH) are censused. We stipulated that for plots to be suitable for analysing tree mortality in response to local conspecific density, they should be at least a few hectares in size with at least two censuses available (that is, longitudinal data on individual trees). The plots for which we obtained data vary in size between 6 ha and 52 ha (Supplementary Table 1), with between 9,718 and 495,577 mapped tree individuals at each site. Censuses have been performed with remeasurement intervals of approximately five years (Supplementary Table 1). The census data collected for each individual include species identity, DBH, spatial coordinates and status (alive or dead).
For the mortality analyses, we selected observations of all living trees of non-fern and non-palm species with DBH < 10 cm in one census and follow-up data in a consecutive census (Extended Data Table 1). We then statistically analysed how tree mortality (measured by the status ‘dead’ or ‘alive’ in the consecutive census) depends on local conspecific density and potential confounders of this relationship (see ‘Species-site-specific mortality models’). We focused on saplings (small trees between 1 cm and 10 cm DBH), on the assumption that CNDD effects are most pronounced in earlier life stages52,53.
For tree individuals with more than one stem, the individual was considered ‘alive’ if at least one of the stems was alive and ‘dead’ if all stems were dead. The DBH of multi-stem trees was calculated from the summed basal area of all stems. For trees with multiple stems at different coordinates, coordinates of the main stem were used. For the forest site Pasoh, where every stem was treated as an individual (information on which stems belong to the same tree was unavailable), we used observations of individual stems.
Observations of trees or stems were excluded when information on coordinates, species, status or date of measurement was missing. Individuals classified as morphospecies were kept and analysed as the respective morphospecies. Status assignments were checked for plausibility and corrected if necessary (for example, trees found to be alive after being recorded as dead in a previous census were set to ‘alive’). If trees or stems changed their coordinates or species between censuses, the most recent information was used.
Definition of local conspecific density
Most previous CNDD studies3,32 have estimated separate effects for CNDD and HNDD. In the context of the Janzen–Connell hypothesis, in which CNDD is a promoter of species diversity, however, we are interested mainly in the difference between CNDD and HNDD, because only a detrimental effect of neighbouring conspecifics that exceeds the effect of any kind of neighbour (that is, irrespective of its species identity) can lead to a stabilizing effect at the population level6,20. We refer to this effect, that is, to the difference between CNDD and HNDD, as ‘stabilizing CNDD’. This effect is more appropriate when estimating the degree of self-limitation for a tree species.
Because CNDD and HNDD are both estimated with uncertainty (characterized by the standard error), previous analyses that separately estimated CNDD and HNDD often faced challenges when formally testing whether conspecific effects are significantly more negative than are heterospecific effects25. Here, we circumvent this problem by estimating the effect of conspecific density, adjusted (in a multiple regression) for total tree density, which is the sum of conspecific and heterospecific density54. Defined in this way, the estimated effect (slope) for conspecific density in the regression corresponds to the effect of CNDD minus HNDD in previous studies55,56 (for details, see Supplementary Methods).
Local conspecific and total densities around each focal tree were calculated as the number of neighbouring trees (N) or their basal area (BA) at the census preceding the census at which tree status was modelled. We considered neighbouring trees of all sizes at distances up to 30 m54 and discarded focal trees that were within 30 m of the plot boundaries. A decrease of neighbourhood effects with increasing distance was considered using two alternative decay functions:
$$\begin{array}{cc}{\rm{exponential}}: & f\left({d}_{k}\right)={e}^{-\frac{1}{\mu }{d}_{k}}\end{array}$$
$$\begin{array}{cc}{\rm{exponential-normal}}: & f\left({d}_{k}\right)={e}^{-\frac{1}{{\mu }^{2}}{{d}_{k}}^{2}}\end{array}$$
with dk being the distance between a focal tree and its neighbour k, and the distance decay parameter μ defining how far neighbourhood effects extend on average.
The estimator for local density (N or BA), the shape of the decay kernel (exponential or exponential–normal) and its parameter μ were optimized through a grid search, optimizing the fit of the mortality models (see next section). The parameter μ was optimized jointly for all species but separately for conspecific and total densities following the idea that the two effects are caused by different agents and thus may act at different spatial scales. We tested all four combinations of density definitions (N or BA, with exponential or normal distance decay) varying μ between 1 and 25 m in 2- m steps. Our selection criterion was the sum of the log likelihood (LL), calculated using the set of species for which all models converged (nspecies = 2,500). The highest overall LL was achieved when local densities were measured as BA with an exponential distance decay and μ = 3 and 17 for conspecific and total density, respectively (Supplementary Fig. 2). This definition of local densities also resulted in an average area under the curve (AUC) comparable with the overall AUC optimum (0.68; difference = 0.001). To ensure that the joint optimization of μ for all species did not induce a bias that correlated with the main predictors, that is, latitude and species abundance, we further examined species-specific optima of μ for those species for which the grid search yielded a distinct optimum of the log likelihood. We found no pattern with respect to latitude and species abundance (Supplementary Fig. 3), justifying the use of a joint optimization.
Species-site-specific mortality models
We used binomial generalized linear mixed models (GLMMs) with a complementary log-log (cloglog) link to model the tree status (‘dead’ or ‘alive’) as a function of conspecific density conD, total density totD and tree size DBH, which were added as potential confounder or precision covariates57. The advantage of the cloglog link over the more traditional logit link is that the cloglog allows better accounting for differences in observation time Δt (see Supplementary Table 1) through an offset term58.
Because evidence suggests that CNDD could be nonlinear and in particular saturating10,25, we used generalized additive models (GAMs) with thin plate splines59 to allow for flexible nonlinear responses of all predictors. When the observations covered more than one census interval, ‘census’ was included as a random intercept. In sum, we model the status Yij of observation i in census interval j as a binomial random variable \({Y}_{ij} \sim {\rm{B}}{\rm{i}}{\rm{n}}{\rm{o}}{\rm{m}}(Pr(\,{y}_{ij}=1))\), where
$$\begin{array}{c}{\rm{l}}{\rm{o}}{\rm{g}}(-{\rm{l}}{\rm{o}}{\rm{g}}(1-Pr(\,{y}_{ij}=1)))={\beta }_{0}+{f}_{{\rm{c}}{\rm{o}}{\rm{n}}{\rm{D}}}({x}_{{\rm{c}}{\rm{o}}{\rm{n}}{\rm{D}}})+{f}_{{\rm{t}}{\rm{o}}{\rm{t}}{\rm{D}}}({x}_{{\rm{t}}{\rm{o}}{\rm{t}}{\rm{D}}})\\ \,\,\,\,\,\,\,\,\,\,+{f}_{{\rm{D}}{\rm{B}}{\rm{H}}}({x}_{{\rm{D}}{\rm{B}}{\rm{H}}})+{u}_{j}+\log (\Delta t)\end{array}$$
Here, Pr(yij = 1) is the mortality probability of observation i in census interval j, fk is the smooth function of the predictor xk, conD, totD and DBH are the predictor variables, β0 is the intercept term, uj is the random intercept for census interval j with \({u}_{j} \sim N(0,{\sigma }_{u}^{2})\) and ∆t is the census interval length in years.
GAM smoothness selection was performed using restricted maximum likelihood estimation (REML). Basis dimensions of smoothing splines were kept at modest levels (k = 10) but were reduced when the number of unique values (nvals) in a predictor was less than 10 (k = nvals – 2). Models were fitted with the function gam() from the package mgcv60 (v.1.8-40).
In this set-up, we fitted species-site-specific mortality models for all species that had at least 20 alive and dead status observations each and at least 4 unique conspecific density values with a range that included the value used to calculate average marginal effects (see ‘Quantification of conspecific density dependence’). The species that did not fulfil these criteria and those for which no convergence was achieved (overall 63.2% of the species) were fitted jointly in one of two groups—rare shrub species and rare tree species (Extended Data Table 1)—following the assumption that different growth forms may differ in their base mortality rate. This allows us to at least consider very rare species for our analyses, even if these species do not contribute to the results to the same extent as species with more observations do. The growth form of each tree species (‘shrub’ or ‘tree’) was derived from a species’ maximum tree size. If the maximum of the average DBH of the six largest trees or stems of each species per census was more than 10 cm, a species was considered a tree, and otherwise it was considered a shrub61,62.
Quantification of conspecific density dependence
On the basis of the species-site-specific mortality models, we then quantified how a change in conspecific density affects mortality probability. The challenge here is that the nonlinear link in the GLMMs implies that effects at the scale of the linear predictor can translate nonlinearly to the response scale (mortality rates) when the estimated intercept differs between individual species and sites31. To obtain an estimate of the strength of stabilizing CNDD that is nonetheless comparable among species and sites, we calculated the average marginal effect (AME) of a small perturbation of conspecific density on mortality probability63 at the response scale. We derived both absolute and relative AME (aAME and rAME, respectively), which can be interpreted as the average absolute (% per year) and relative (%) change, respectively, in mortality probability caused by the increase in conspecific density. In meta-analysis and econometrics, aAME is also known as the average risk difference, and rAME + 1 as the average risk ratio64,65.
To obtain aAME and rAME, we first calculated the absolute and relative effect of one additional conspecific neighbour on the mortality probability (response scale) for each observation i:
$${{\rm{aME}}}_{i}={p}_{i,{{\rm{conD}}}_{i}+1}-{p}_{i,{{\rm{conD}}}_{i}}$$
$${{\rm{rME}}}_{i}=\frac{{p}_{i,{{\rm{conD}}}_{i}+1}}{{p}_{i,{{\rm{conD}}}_{i}}}-1=\frac{{p}_{i,{{\rm{conD}}}_{i}+1}-{p}_{i,{{\rm{conD}}}_{i}}}{{p}_{i,{{\rm{conD}}}_{i}}}$$
Here, pi is the mortality probability at the response scale and conDi is the observed local conspecific density. The subscript conDi + 1 denotes the new conspecific density, which is obtained by adding one conspecific neighbour with DBH = 2 cm at a one-metre distance, a relatively small perturbation that was within the range of observed conspecific densities even for rare species. A larger perturbation in conspecific densities could create extrapolation problems. For each observation, aMEi and rMEi were calculated using observed conspecific densities. Likewise, confounders—that is, total density, DBH and census interval—were kept at observed values, and the interval length was fixed at one year. As an alternative quantification of density dependence that links to theoretical considerations from coexistence theory7 (invasion criterion35), we quantified CNDD at low conspecific densities by setting conDi = 0 and again increasing it by one additional conspecific neighbour with DBH = 2 cm at a one-metre distance. As a further alternative, we calculated CNDD as the change in mortality resulting from a change in conspecific density from the first to the third quantile of observed conspecific densities per species to estimate how important CNDD is effectively for small tree mortality. It must be noted that values from this latter metric should not be compared between species (or sites), because the change in conspecific density is different for each species and tends to increase with species abundance.
Individual marginal effects (aMEi and rMEi) were averaged over all observations per species to obtain average marginal effects31. Because there is no analytical function to forward the uncertainty of the GAM predictions to the response scale, we estimated uncertainties; that is, sampling variances vlm, and significance levels for species-site-specific aAME and rAME by simulation. To this end, we simulated 500 sets of new model coefficients from a multivariate normal distribution with the unconditional covariance matrix of the fitted model, calculated aAME and rAME for each set66 and used quantiles of the simulated distributions to approximate sampling variances and significance levels of CNDD estimates.
In our results, we concentrate our discussion on rAME because we consider relative changes in mortality to be ecologically more meaningful than absolute changes. The reason is that the relevance of an increase in mortality for a species’ fitness strongly depends on its base mortality rate. Vice versa, if CNDD effects exist, it is to be expected that they are higher in absolute terms for species that already have higher absolute mortality rates. Moreover, given that species-specific mortality rates may also correlate with species abundance and latitude, the use of absolute mortality rates is likely to be more prone to confounding. To be comparable with previous studies, which commonly use absolute effects, results for the two main meta-regressions are also presented for the absolute effects; that is, aAME estimates (Extended Data Fig. 4 and Extended Data Table 3).
Meta-regressions for CNDD patterns
To test for latitudinal patterns in stabilizing CNDD, we fitted meta-regressions34,67 using the species-site-specific CNDD estimates. The advantage of these models is that they simultaneously account for the uncertainties in aAME and rAME estimates (sampling variances)—much like measurement error models—as well as heterogeneity among sites and species through a multilevel model:
$${{\rm{AME}}}_{lm}={b}_{0}+{r}_{l}+{s}_{lm}+{e}_{lm}+f({\rm{predictors}})$$
$${r}_{l} \sim N\left(0,{\sigma }_{r}^{2}\right)$$
$${s}_{lm} \sim N\left(0,{\sigma }_{s}^{2}\right)$$
$${e}_{lm} \sim N\left(0,{v}_{lm}\right)$$
Here, AMElm is the average marginal effect for site l and species m, b0 is the intercept, rl is the random effect for site l (normally distributed with \({\sigma }_{r}^{2}\)), slm is the random effect of species m (normally distributed with \({\sigma }_{s}^{2}\)) and elm is the uncertainty of the individual estimates (normally distributed with the species-site-specific sampling variance vlm). Omitting the random effects would lead to inappropriate estimates because it does not consider the true interspecific variation in species’ CNDD. To improve the normality assumption of the residuals of the meta-regressions, rAMEs were log-transformed after adding 1 before calculating the sampling variances (see above); aAME remained untransformed.
Depending on the respective prediction to be evaluated, we used different meta-regression models. To evaluate latitudinal patterns in average CNDD and in the association of CNDD and abundance, we fitted multilevel models to all species-site-specific estimates (see model formula above): the first including absolute latitude as a predictor (Fig. 2 and Table 1a) and the second also including log-transformed species abundance and its interaction with latitude (Fig. 3 and Table 1b).
Absolute latitude was calculated as the distance (in degrees) to the equator. This metric does not distinguish between the northern and southern hemispheres and is commonly used as a proxy for the current and past bio-climatic variables that are assumed to underlie most latitudinal biological patterns68,69. We calculated the abundance of each tree species per site as the number of all living trees (or stems, for the Pasoh site) with DBH ≥ 1 cm per hectare on the entire plot. Abundance for the two groups of rare species (rare trees and rare shrubs) was calculated as the average of species abundances within the respective group. The predictors were centred at abundance = 1 tree per hectare and absolute latitude = 11.75°, so that main effects reflect slopes and respective significance tests for rare tropical species (Table 1).
We also separately fitted meta-regressions for each site with species as a random intercept: first, without any predictor to obtain mean CNDD and its s.d. among species per site (Figs. 2 and 4); and then with species abundance as a predictor to illustrate site-specific relationships of CNDD and abundance (Fig. 1).
AMEs calculated for species-specific interquantile ranges were aggregated in a global meta-regression with random intercepts for sites and species within sites to obtain a global average of CNDD and assess its importance for small tree mortality (Extended Data Fig. 1).
Models were fitted with REML using the functions rma.mv() and rma() from the package metafor70 (v.3.4-0) for the global and site-specific cases, respectively.
Robustness tests
Statistical assumptions of the mortality models were verified on the basis of simulated residuals generated with the package DHARMa (v.0.4.6)71. Distributional assumptions and residual patterns against predictors were assessed visually, revealing no critical violations of assumptions and a consistently good model fit. To verify that no additional unobserved local confounders, particularly habitat effects, were affecting the relationship between conspecific density and mortality, we tested each mortality model for spatial autocorrelation using the package DHARMa (ref. 71). After adjusting P values for multiple testing using the Holm method, significant spatial autocorrelation was detected in only seven models, or 0.28% of all species–site combinations, which means that there is no indication that local species-specific CNDD estimates were affected by spatial pseudo-replication.
Model diagnostics for the meta-regressions were based on standardized residuals and visual assessments. Because of the unbalanced design (more tropical than temperate species; see Supplementary Fig. 1c), we performed additional robustness tests by identifying influential species-site-specific CNDD estimates and refitting the two main meta-regression models (see Table 1) with a reduced dataset without these observations. We removed 99 CNDD estimates that had Cook’s distances larger than 0.005 in the abundance-mediated CNDD model72. Meta-regressions fitted with these reduced datasets revealed similar patterns and significance levels (Extended Data Fig. 3 and Extended Data Table 2).
To evaluate the robustness of the entire analysis pipeline with respect to potential abundance- and latitude-related biases11,12, we repeated all steps of the analysis (mortality models, average marginal effects and meta-regressions) with two randomizations of the original dataset (similar tests highlighted biases in a previously described pipeline8, see also refs. 11,12). We randomized (1) observations of tree status within each species, thus removing any relationship between mortality and predictors but maintaining species-level mortality rates; and (2) observations of local conspecific density within each species, thus removing the relationship between mortality and conspecific density but maintaining the relationships between mortality and confounders. Meta-regressions applied to these randomized datasets revealed close to zero CNDD and no considerable patterns with latitude or species abundance (Extended Data Fig. 2 and Extended Data Table 2). When randomizing tree status, rare species exhibited minimally, but significantly, stronger CNDD, but the effect sizes varied by orders of magnitude from those observed in the original dataset. We therefore consider our results robust to statistical artefacts related to species abundance and latitude.
In addition, not only statistical biases but also alternative explanations could create a spurious correlation between CNDD and species abundance. To test this, we included potential confounders for this relationship in the ‘abundance-mediated CNDD’ model. Following the idea that fast-growing tree species with short life spans (that is, lower survival rates) tend to be rarer43—a pattern also observed across the 23 forest sites analysed here (Supplementary Fig. 1a,b)—and at the same time may experience stronger CNDD41, we considered two sets of predictors that are proxies for different life history strategies, namely: (1) species-specific growth and survival rates; and (2) species-specific values along two demographic trade-off axes73,74. Species-specific growth was calculated as the median of the annual DBH increment, log-transformed after adding 1. For survival, we calculated mean annual survival rates (based on the intercept of a GLM similar to the mortality models for CNDD but without predictors) and applied a logit-transformation. Both rates were standardized within sites (that is, subtracting the mean and dividing by the s.d.) to account for differences in the realized demographic spectrum between sites. The demographic trade-offs reflect the two axes ‘growth–survival’ and ‘stature–recruitment’ and were adapted from a procedure described previously73 using species-specific growth and survival rates (as described before) and the species’ maximum size (stature), calculated as the log-transformed 90th percentile of the DBH, again standardized within sites. In both cases, we included main effects of the two predictors and their interaction. Accounting for life history strategies did not change the patterns obtained, and species abundance and CNDD were still strongly and statistically significantly correlated in tropical forests (Extended Data Table 4).
Stable coexistence and interspecific variation in CNDD
If CNDD varies strongly among species and the resulting interspecific fitness differences are not compensated by equalizing mechanisms6,33, the stabilizing advantage of CNDD may not promote diversity. One study14 suggested, on the basis of simulations, that the number of species maintained strongly drops when the coefficient of variation (CV = s.d./mean) for CNDD is above 0.4 (see the second figure in that study); that is, the stronger CNDD becomes, the more interspecific variation it enables. Similarly, another study15 found considerably fewer species with increasing standard deviations of CNDD supporting a comparable threshold of CV = 0.4 (s.d. = 0.2 at mean CNDD = 0.5; see the second figure in that study). Another study75, which also investigated the effect of interspecific variation in CNDD, identified no threshold for stable coexistence, which is most likely to be caused by the relatively small variation in CNDD that this study tested (see the second figure in that study). Although it is not entirely clear whether the threshold of CV = 0.4 is truly due to the magnitude of fitness differences or to the fact that some species tend to have almost no CNDD when interspecific variation becomes large, the consistency of this threshold, despite different implementations of CNDD14,15, provides a starting point for evaluating the relevance of CNDD for community assembly. We estimated true interspecific variation of CNDD within forest communities fitting site-specific meta-regressions without predictors (see ‘Meta-regressions for CNDD patterns’), which are particularly helpful in this case because the raw variability of species-specific CNDD estimates is also driven by statistical uncertainty.
Reporting summary
Further information on research design is available in the Nature Portfolio Reporting Summary linked to this article.
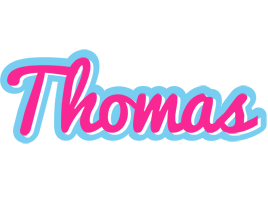
Dr. Thomas Hughes is a UK-based scientist and science communicator who makes complex topics accessible to readers. His articles explore breakthroughs in various scientific disciplines, from space exploration to cutting-edge research.