Summary: A new study combines deep learning with neural activity data from mice to unlock the mystery of how they navigate their environment.
By analyzing the firing patterns of “head direction” neurons and “grid cells,” researchers can now accurately predict a mouse’s location and orientation, shedding light on the complex brain functions involved in navigation. This method, developed in collaboration with the US Army Research Laboratory, represents a significant leap forward in understanding spatial awareness and could revolutionize autonomous navigation in AI systems.
The findings highlight the potential for integrating biological insights into artificial intelligence to enhance machine navigation without relying on GPS technology.
Key Facts:
- Deep Learning Decodes Navigation: Researchers used a deep learning model to decode mouse neural activity, accurately predicting a mouse’s location and orientation based solely on the firing patterns of “head direction” neurons and “grid cells.”
- Collaboration with US Army Research Laboratory: The study was conducted in collaboration with the US Army Research Laboratory, aiming to integrate biological insights with machine learning to improve autonomous navigation in intelligent systems without GPS.
- Potential for AI Systems: The findings could inform the design of AI systems capable of navigating autonomously in unknown environments, leveraging the neural mechanisms underlying spatial awareness and navigation found in biological systems.
Source: Cell Press
Researchers have paired a deep learning model with experimental data to “decode” mouse neural activity.
Using the method, they can accurately determine where a mouse is located within an open environment and which direction it is facing just by looking at its neural firing patterns.
Being able to decode neural activity could provide insight into the function and behavior of individual neurons or even entire brain regions.
These findings, publishing February 22 in Biophysical Journal, could also inform the design of intelligent machines that currently struggle to navigate autonomously.
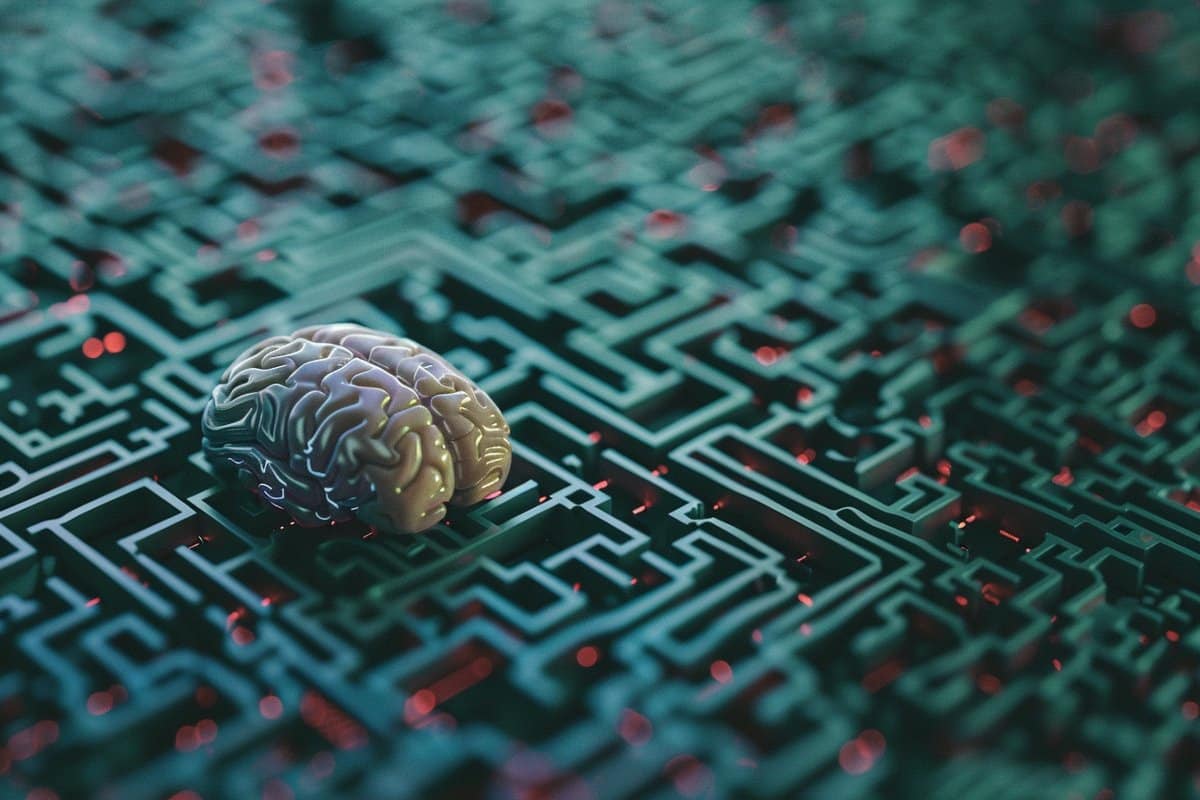
In collaboration with researchers at the US Army Research Laboratory, senior author Vasileios Maroulas’ team used a deep learning model to investigate two types of neurons that are involved in navigation: “head direction” neurons, which encode information about which direction the animal is facing, and “grid cells,” which encode two-dimensional information about the animal’s location within its spatial environment.
“Current intelligence systems have proved to be excellent at pattern recognition, but when it comes to navigation, these same so-called intelligence systems don’t perform very well without GPS coordinates or something else to guide the process,” says Maroulas, a mathematician at the University of Tennessee Knoxville.
“I think the next step forward for artificial intelligence systems is to integrate biological information with existing machine-learning methods.”
Unlike previous studies that have tried to understand grid cell behavior, the team based their method on experimental rather than simulated data.
The data, which were collected as part of a previous study, consisted of neural firing patterns that were collected via internal probes, paired with “ground-truthing” video footage about the mouse’s actual location, head position, and movements as they explored an open environment.
The analysis involved integrating activity patterns across groups of head direction and grid cells.
“Understanding and representing these neural structures requires mathematical models that describe higher-order connectivity—meaning, I don’t want to understand how one neuron activates another neuron, but rather, I want to understand how groups and teams of neurons behave,” says Maroulas.
Using the new method, the researchers were able to predict mouse location and head direction with greater accuracy than previously described methods. Next, they plan to incorporate information from other types of neurons that are involved in navigation and to analyze more complex patterns.
Ultimately, the researchers hope their method will help design intelligent machines that can navigate in unfamiliar environments without using GPS or satellite information. “The end goal is to harness this information to develop a machine-learning architecture that would be able to successfully navigate unknown terrain autonomously and without GPS or satellite guidance,” says Maroulas.
About this neuroscience research news
Author: Kristopher Benke
Source: Cell Press
Contact: Kristopher Benke – Cell Press
Image: The image is credited to Neuroscience News
Original Research: Open access.
“A Topological Deep Learning Framework for Neural Spike Decoding” by Vasileios Maroulas et al. Biophysical Journal
Abstract
A Topological Deep Learning Framework for Neural Spike Decoding
The brain’s spatial orientation system uses different neuron ensembles to aid in environment-based navigation. Two of the ways brains encode spatial information are through head direction cells and grid cells. Brains use head direction cells to determine orientation, whereas grid cells consist of layers of decked neurons that overlay to provide environment-based navigation.
These neurons fire in ensembles where several neurons fire at once to activate a single head direction or grid. We want to capture this firing structure and use it to decode head direction and animal location from head direction and grid cell activity.
Understanding, representing, and decoding these neural structures require models that encompass higher-order connectivity, more than the one-dimensional connectivity that traditional graph-based models provide.
To that end, in this work, we develop a topological deep learning framework for neural spike train decoding. Our framework combines unsupervised simplicial complex discovery with the power of deep learning via a new architecture we develop herein called a simplicial convolutional recurrent neural network.
Simplicial complexes, topological spaces that use not only vertices and edges but also higher-dimensional objects, naturally generalize graphs and capture more than just pairwise relationships.
Additionally, this approach does not require prior knowledge of the neural activity beyond spike counts, which removes the need for similarity measurements.
The effectiveness and versatility of the simplicial convolutional neural network is demonstrated on head direction and trajectory prediction via head direction and grid cell datasets.
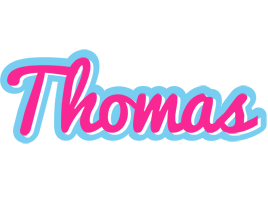
Dr. Thomas Hughes is a UK-based scientist and science communicator who makes complex topics accessible to readers. His articles explore breakthroughs in various scientific disciplines, from space exploration to cutting-edge research.